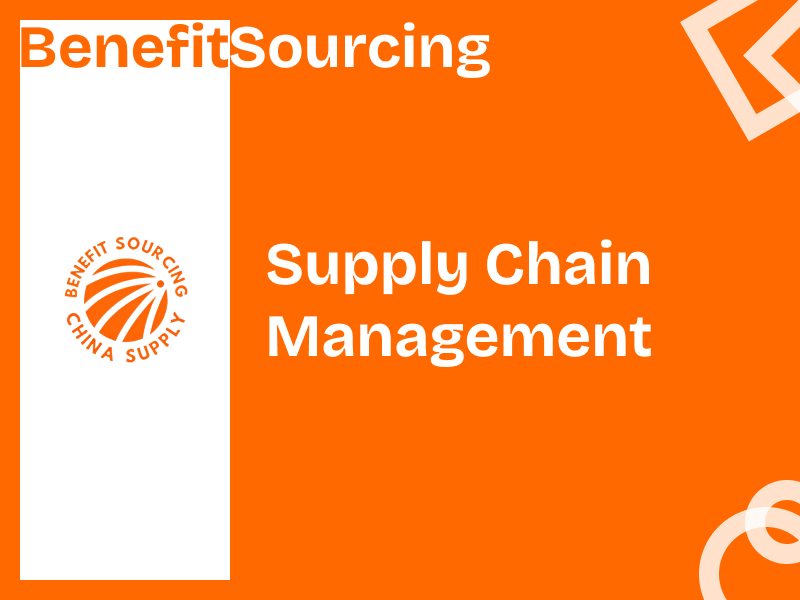
Leveraging Data Analytics for Supply Chain Optimization
For small and medium-sized businesses (SMBs), retailers, and e-commerce sellers sourcing products internationally, supply chain optimization is critical to reducing costs, improving efficiency, and staying competitive. Data analytics provides actionable insights to address common challenges like demand forecasting inaccuracies, inventory mismanagement, and logistical bottlenecks. Below are practical strategies to harness data-driven approaches for supply chain improvement.
1. Data Collection and Integration
Effective supply chain management starts with consolidating data from multiple sources:
- IoT devices and sensors: Capture real-time data on inventory levels, warehouse conditions, and shipment tracking.
- Sales platforms: Integrate e-commerce and point-of-sale (POS) systems to track customer demand patterns.
- Supplier performance metrics: Monitor delivery times, quality compliance, and cost fluctuations.
- External factors: Incorporate market trends, weather data, and geopolitical events that may disrupt logistics.
Centralizing this data into a unified platform (e.g., cloud-based ERP systems) ensures visibility across the entire supply chain.
2. Demand Forecasting with Predictive Analytics
Accurate demand prediction minimizes overstocking or stockouts. Machine learning algorithms analyze historical sales data, seasonality, and promotional impacts to forecast future needs. For example:
- Time-series analysis: Identifies recurring patterns, such as holiday sales spikes or seasonal dips.
- Sentiment analysis: Leverages social media and customer reviews to gauge emerging trends.
- Collaborative filtering: Recommends products based on purchasing behavior similarities among customer segments.
Businesses using predictive models have reported up to 30% reductions in inventory costs and improved order fulfillment rates.
3. Inventory Optimization
Real-time analytics enables dynamic inventory management:
- ABC analysis: Classifies inventory based on value and turnover rates (e.g., high-value items requiring tight control vs. low-priority stock).
- Safety stock calculation: Uses variability in demand and lead times to determine optimal buffer stock levels.
- Automated reorder triggers: Alerts managers when stock dips below predefined thresholds.
Retailers adopting these strategies often achieve 15–25% improvements in inventory turnover ratios.
4. Logistics and Route Optimization
Data-driven logistics reduce transportation costs and delivery delays:
- Route planning algorithms: Factor in traffic, fuel costs, and carrier availability to determine the fastest, cheapest routes.
- Multi-modal optimization: Evaluates combinations of shipping methods (air, sea, rail) to balance speed and cost.
- Carrier performance tracking: Identifies reliable partners using on-time delivery rates and damage reports.
Companies using AI-powered logistics tools have cut transportation expenses by 10–20% while improving delivery accuracy.
Analytics helps evaluate and strengthen supplier relationships:
- Scorecards: Rate suppliers on quality, lead times, and compliance using weighted KPIs.
- Risk assessment: Analyzes financial stability, geopolitical factors, and environmental compliance to mitigate disruptions.
- Collaborative forecasting: Shares demand forecasts with suppliers to align production schedules.
Businesses that prioritize data-backed supplier selection reduce procurement lead times by 25–35%.
6. Real-Time Monitoring and Risk Mitigation
Proactive monitoring prevents costly disruptions:
- IoT-enabled tracking: Monitors shipments for temperature deviations (e.g., perishable goods) or delays.
- Predictive maintenance: Analyzes equipment sensor data to schedule repairs before breakdowns occur.
- Scenario modeling: Simulates disruptions (e.g., port closures) to develop contingency plans.
Cost-effective technologies make advanced analytics accessible:
- Cloud-based analytics platforms: Offer pay-as-you-go models for inventory and demand forecasting.
- Low-code/no-code solutions: Enable non-technical teams to build dashboards for real-time insights.
- Blockchain: Enhances transparency in supplier contracts and product traceability.
For instance, small e-commerce sellers using cloud ERP systems report 40% faster decision-making and 20% lower operational costs.
Key Challenges and Solutions
- Data quality: Implement automated cleansing tools to address inconsistencies.
- Skill gaps: Partner with third-party analytics providers or invest in user-friendly platforms.
- Integration costs: Start with modular solutions focused on high-impact areas (e.g., inventory or demand forecasting).
By systematically applying data analytics, businesses can transform supply chain complexities into competitive advantages—whether through smarter inventory decisions, resilient logistics, or stronger supplier partnerships.